Written by: Danila Stepanenkov
How are people pricing AI in the software industry?
Around the same time last year, companies were mostly talking about AI but not monetizing it—either giving it away for free or charging an add-on price with existing services (priced per seat).
Today, how AI is integrated into software has changed, partly because of the R&D costs and operational expenses associated with the technology, which drive pressure for companies to monetize and find revenue growth. This essentially adds additional items to the “Revenue” header on an income statement, alongside the typical “product” and “service” revenue lines.
Traditional SaaS products are built for productivity and collaboration, such as workflows with per-seat and subscription pricing. As more employees use these software tools, the overall productivity of the company scales. This justifies the “per seat” pricing model, where the number of seats strengthens the value of the software. On the other hand, AI products are usually designed for automation, meaning pricing does not scale as more people log in. These products take over the collective repetitive tasks, rather than serving individual productivity. This suggests that usage-based pricing, such as charging per processed data unit, allows companies to recover costs more directly.
The way to monetize AI looks different than before. Overall, it makes more sense to charge for the work delivered by the AI, rather than the number of people who have access to it. This is a key difference between recurring revenue and re-occurring revenue. Consider an Amazon subscription, where customers are billed monthly like clockwork, with no second thoughts—that’s recurring revenue. Now consider Uber rides, payment systems like Toast where restaurants rely on the service but revenue varies, or Fiverr, where demand for contractors is unpredictable—that’s re-occurring revenue. AI products seem to fall into this re-occurring category, where customers might continue using the service, but the revenue is not as predictable.
Usually, software products capture 10-20% of the economic value they deliver. For example, if a CRM tool helps a business improve sales revenue by $100,000, that software typically costs around $10,000 - $20,000. However, if you can price based on the usage/outcome of the AI product, you can capture more, such as 20-40% of the economic value. For instance, if an AI tool reduces operational costs or increases revenue by $100,000, the company could charge $20,000 - $40,000, likely exceeding a fixed price. This approach also increases pricing power because customers only pay a share of the ROI, minimizing risk by avoiding large upfront costs.
Units of work completed vs. access to software
Seat-based pricing means customers can use the product as much as they want for a fixed per-seat fee. The ROI depends on how effectively the business uses the software, which often leads to shelfware.
In terms of valuation, software multiples are closely tied to ARR, unlike other industries that focus on EBITDA or EBIT. ARR is attractive in the software industry because once a product is built and deployed, the associated costs are primarily CAC. AI products, however, often require more computational resources like GPUs and cloud infrastructure, which increase costs and reduce margins, sometimes resulting in negative margins.
Is it time for a new metric?
When valuing AI companies today, a metric that accounts for the multiple revenue streams, each with different costs, margin profiles, and qualities of income, might be necessary. Especially in a DCF analysis, factoring in gross margins can provide more accurate cash flow projections and discount rates.
Take this case-use article here posted a week ago about how to think of this new wave of revenue with AI products.
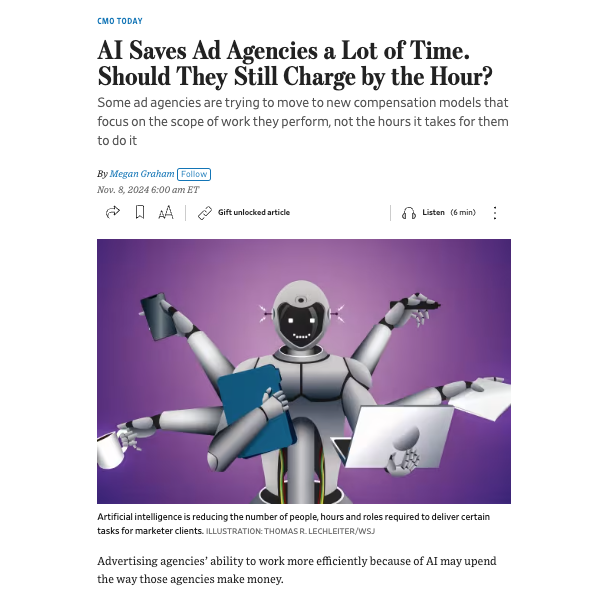
Now the question is, how do investment bankers working with these “TargetCo’s” in M&A deals actually value these companies with AI products?
ServiceTitan Case Study
ServiceTitan, a cloud-based end-to-end software company in the trade industry, shows us a perfect example of the "usage-based" outcome model talked about in this article.
ServiceTitan is different from enterprise software companies because rather than providing specific functional workflows, the company targets a specific customer, and provides entire end-to-end services, and covers all of their workflows. This makes ServiceTitan’s business model more sticky since customers rely on multiple critical functions. This also allows for ServiceTitan to have AI affect the product as a whole, in multiple areas, like the scheduling of a dispatched trade-expert and GPS of the expert, to collecting the collecting of revenue, to collectively managing the employees.
This will also allow for ServiceTitan to leverage a massive data asset to train their AI models on, since their products are being used all-day, every-day. This is something that might be noticeably more prominent in technology, where Enterprise Software companies try to build entire end-to-end cloud-based products, rather than just providing functional workflows, to harvest more data.
Companies like ServiceTitan will have huge differentiating data moats with their end-to-end services that will allow them to optimize operations, innovate and develop product faster; deliver new AI-based capabilities at much larger scale than traditional legacy software systems that provide functional workflows.
Why this is relevant to the article
ServiceTitan has a feature called a "TitanAdvisor Score," displayed on a dashboard for each client. This dashboard measures platform utilization, showing that customers in the top TitanAdvisor quartile experience median YoY gross transaction volume (GTV) growth of 20-25%, while those in the bottom quartile experience 8% growth. This reinforces the idea that increased utilization and investment in ServiceTitan lead to higher revenue and greater business success.
This re-occurring revenue adds complexity because it is less predictable and heavily reliant on customer adoption and demand. If, in the future, ServiceTitan were acquired by a large enterprise software company or taken private by a sponsor, investment bankers might need to develop a new metric to measure revenue accurately.
One potential idea is "Revenue Per Unit of Work." With TitanAdvisor showing a strong correlation between platform usage and GTV growth, a usage-based metric like Revenue Per GB of Data Processed or Revenue Per Task Automated could more precisely quantify the platform’s value.
Certain intricacies like these are what CFO's at companies like ServiceTitan might need to think about. With AI products and tools becoming more popular, it's more likely that CFO's will have double the amount of things to think about and work to do, rather than being replaced by AI.
Comments